Research output today is faster, more refined, and visually polished in ways that suggest meaningful progress. Presentations arrive on time, the language is articulate, and AI tools appear to be delivering exactly what they promise.
Yet beneath this surface-level improvement, something more essential is quietly being lost. The critical thinking that once gave research its strategic value is fading, replaced by a reliance on fluency and form. While the work may still look professional, the depth that once supported confident decision-making is becoming harder to find.
This is not a theoretical concern about what might happen in the future. It is already reshaping how choices are framed, how insight is interpreted, and how decisively leaders are able to act.
Insight is being replaced by output
The rise of AI has introduced speed and consistency into research workflows. Teams are producing content faster, summarizing data more efficiently, and delivering professional-looking deliverables at scale. However, the very convenience of these tools is encouraging a subtle disengagement from the deeper analytical work that once distinguished strong research teams.
A 2025 study in Societies1 found that professionals who frequently rely on generative AI are significantly less likely to engage in critical evaluation. The problem is not that AI gives wrong answers. It is that users become less inclined to question what they receive. Instead of challenging assumptions or exploring alternative perspectives, teams are beginning to accept outputs as final. The result is a research function that moves quickly but thinks less.
Everyone sounds smart. No one sounds different.
As more organizations adopt the same public AI tools trained on similar datasets, the insights generated through them are becoming harder to tell apart. Reports start to sound similar. Ideas begin to converge. Strategies look less like distinctive responses to complex problems and more like well-phrased summaries of common knowledge.
A recent study on AI-assisted ideation found that teams using AI early in the creative process produced fewer original ideas and were less likely to challenge foundational assumptions. The absence of tension during early thinking phases led to less diverse conclusions. What appeared to be productivity gains were, in many cases, reductions in intellectual depth.
This shift carries consequences that go beyond aesthetics. What once made a research function valuable was its ability to bring a distinctive perspective and uncover the nuance that others missed. When that edge is dulled, the organization begins to lose a source of strategic advantage.
Zillow didn’t have a model problem; it had a thinking problem.
Zillow’s failed iBuying initiative illustrates what can happen when fluency is mistaken for sound judgment. The company relied heavily on an algorithm to guide large-scale real estate purchases. While the model worked under stable conditions, it failed to adapt when the market shifted. More importantly, no one stepped in to challenge its assumptions or intervene when the data started to misalign with reality.
The outcome was a 300-million-dollar loss and the shutdown of a major strategic initiative. This was not a technical failure. It was a leadership breakdown rooted in overconfidence and under-questioning. That same pattern is now quietly emerging in research teams across industries. Outputs appear reasonable, but the intellectual checks behind them have weakened.
If you don’t fix this, you’ll lose more than accuracy
You will lose trust.
The decline in critical thinking may go unnoticed at first. Research still gets delivered. Presentations still go out. But the quality of insight begins to erode. Leaders start to sense that something is off. They may not point to specific errors, but they feel a lack of depth. They start to second-guess the work, lean on external sources, or rerun analyses through different channels.
Over time, the function loses influence. It becomes a delivery vehicle rather than a driver of strategy. Confidence in its conclusions fades. And when the next complex, high-stakes decision emerges, the team may find itself unprepared, not because of a lack of data, but because it no longer knows how to process ambiguity without help.
High-performing leaders are quietly rebuilding discipline
Forward-thinking research leaders are not rejecting AI. Instead, they are actively reshaping how it is integrated into their teams. They are treating AI-generated output as a starting point rather than a finished product. Teams are being trained to annotate and validate their inputs, to clearly separate machine-generated content from original thought, and to justify conclusions with reference to both.
In practice, this means inserting moments in the workflow where second-order thinking is required. It may involve pausing after the first answer to ask what was missed. It may include deliberately designing work sessions where AI is excluded in order to sharpen human reasoning. And it often requires a shift in how research quality is measured. That shift moves away from speed and polish and focuses instead on clarity, originality, and analytical rigor.
What strong teams should do now
The divide in research capability today is not about who has access to AI and who does not. That gap has already closed. The real divide lies between teams that preserve the habit of thinking and those that have gradually stopped practicing it.
AI will not make your team better or worse. It will simply amplify the patterns already in place. If your team knows how to ask hard questions, challenge assumptions, and refine ideas through debate, AI can accelerate that. If your team accepts whatever the tool returns on the first try, AI will help them move faster in the wrong direction.
This is the moment to reassert what research is supposed to do. That means creating an environment where analysis is challenged before it is shared, where conclusions are earned rather than assembled, and where the goal is not the speed of output but the clarity of understanding.
Leaders who are serious about rebuilding strategic thinking are taking clear steps now:
- They are resetting expectations around what makes a deliverable "done," prioritizing critical review over completion.
- They are building checkpoints into workflows that force second-order thinking before recommendations are presented.
- They are making time for research without tools, not to be contrarian, but to keep analytical instincts active.
- They are defining performance not by how fast the work moves, but by how well it holds up under pressure.
The opportunity is not to reject AI or slow everything down. It is to move forward with more deliberate standards ensuring that speed never comes at the expense of insight.
Teams that get this right will not just look sharper; they will think sharper. In a landscape where so many organizations are optimizing for volume, those who double down on judgment will stand apart.
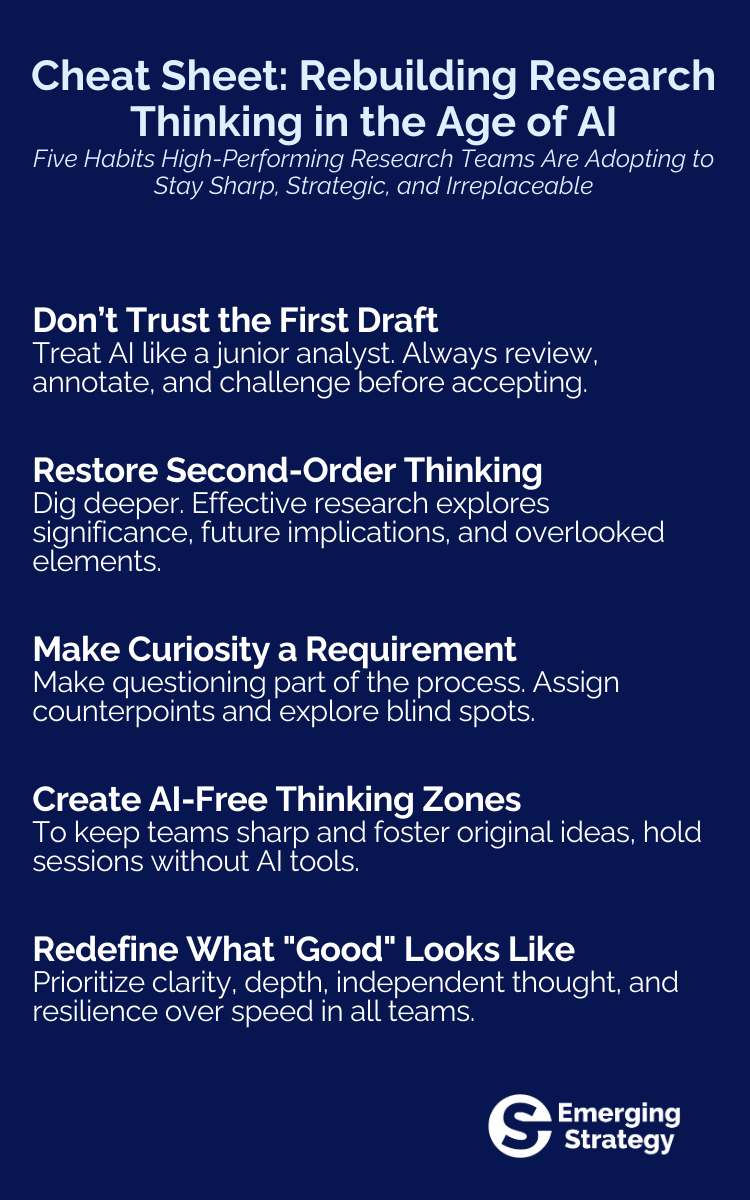