AI has flooded every corner of strategic and market intelligence. Everyone’s talking about it. Everyone’s using it. Everyone thinks they’re keeping up.
But here’s the uncomfortable truth: most companies’ AI adoption in intelligence is making them look more sophisticated while actually deepening their blind spots.
The illusion of insight powered by public AI tools is becoming a strategic liability.
The gap isn’t between companies who use AI and those who don’t, it’s between those who know how to layer AI intelligently and those stuck in the false comfort of generic answers.
Big Companies Are Spending Millions on Proprietary AI: Can You Afford to Compete?
Leading consulting firms like EY and McKinsey have invested heavily in building proprietary AI-driven intelligence platforms. But even with immense financial backing, EY's AI still falls short of the capabilities offered by public models like ChatGPT.
If industry giants, despite their massive resources, can't outpace publicly available AI solutions, consider this:
- Infrastructure costs are astronomical: Developing enterprise-grade AI demands extensive computing power, storage, and security infrastructure.
- Specialized talent is scarce and expensive: Data scientists and AI experts are among the most sought-after professionals, often priced beyond reach for mid-sized or non-tech firms.
- Rapid obsolescence: Proprietary AI solutions require constant updates to remain relevant. Without these resources, your investment could quickly become outdated.
If you aren’t EY or McKinsey, the reality is stark: Building your own AI is simply impractical.
Everyone’s using the same tools, so why do you think you’re getting an edge?
AI adoption is surging at an unprecedented rate. Consider these sobering realities:
- As of 2024, 71% of organizations are regularly using generative AI in at least one business function, up from 33% in 20231.
- AI adoption rose from 55% in 2023 to 72% in 2024, showing a steep year-over-year increase even outside of GenAI-specific use cases.
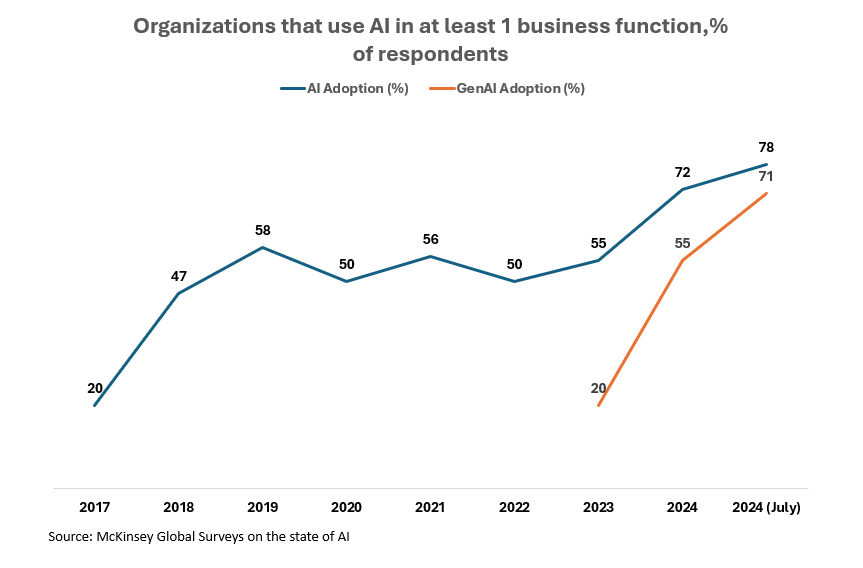
ChatGPT. Claude. Gemini. Every research function has added one (or more) of these tools to their workflow.
And yes, they help you:
- Move faster
- Draft quicker
- Find decent answers on short deadlines
But here’s the strategic problem:
- These models weren’t trained on your market
- They don’t know what your customers care about
- They weren’t built for your competitors, regulators, or product strategy
So when you're relying on them for strategic intelligence, what you're actually doing is outsourcing your judgment to a tool trained on the internet.
That’s not intelligence.
That’s Google with better grammar.
Companies slow to integrate AI aren’t just failing to advance, they're actively losing ground to competitors leveraging AI strategically.
Internal resistance is holding you back more than you realize
Even organizations convinced of AI's importance encounter significant internal hurdles, including:
- Employee fears about job displacement: Many perceive AI as a direct threat to their roles, fueling resistance and skepticism.
- A lack of understanding about AI’s true potential: Without clear communication and proper education, your teams may undervalue or even sabotage AI initiatives.
Resolving these internal barriers requires thoughtful, transparent communication emphasizing AI’s role as an enhancer, not a replacer, of human capability.
Proprietary AI isn’t the answer either, unless you have McKinsey’s budget
Some companies try to build their own AI to “own the edge.”
But unless you're a top-tier firm with $100M+ to burn on infrastructure, data pipelines, and top-tier AI talent, you're going to end up with a slower, worse version of what already exists.
Even EY’s internal AI platform was recently benchmarked as less capable than publicly available GenAI. And that’s EY.
So where does that leave everyone else?
This is the real gap
It’s not between AI vs no AI.
It’s between hype-driven AI use and strategically guided AI use.
What Research Leaders should actually be worried about
Most research and intelligence functions now report higher speed.
But when you dig deeper, they often report:
- Lower confidence in findings
- Greater internal pushback
- An explosion of surface-level insights but a drought of decision-ready intelligence
The shift toward AI-enhanced research should have unlocked new layers of insight.
Instead, many teams have unknowingly replaced depth with output and are walking into boardrooms with beautifully packaged mediocrity.
The fix isn’t a better model. It’s a better strategy
If you want to lead with intelligence in the AI era, you need to flip the script.
- Don’t chase new tools.
Build layered workflows that combine public AI’s speed with custom intelligence, rigorous verification, and strategic nuance. - Don’t assume “faster” means “better.”
Focus on getting the right questions answered—not just getting answers faster. - Don’t let AI replace your team’s judgment.
Train your team to interrogate AI output like they would a junior analyst: useful, but rarely right on the first pass.
The research leaders who will win in this next phase won’t be the ones who automate the most.
It’ll be the ones who design intelligence systems where humans and AI amplify each other without either taking over blindly.
This is the moment to rebuild your intelligence stack
You don't need to build an AI platform.
You don't need to hire a team of PhDs.
You just need to admit: your current tools aren’t enough.
Then, rethink the foundations:
- What does “insight” mean in your context?
- Where can AI truly enhance (vs dilute) your research?
- How do you validate, localize, and challenge what AI gives you?
The intelligence arms race has already started
Most teams don’t realize it until they’ve already lost.
The companies winning right now aren’t the ones with the flashiest AI tools.
They’re the ones quietly reengineering their research, insight, and decision-making processes before the market forces them to.
So the real question is:
Are you building an edge, or just automating your blind spots?